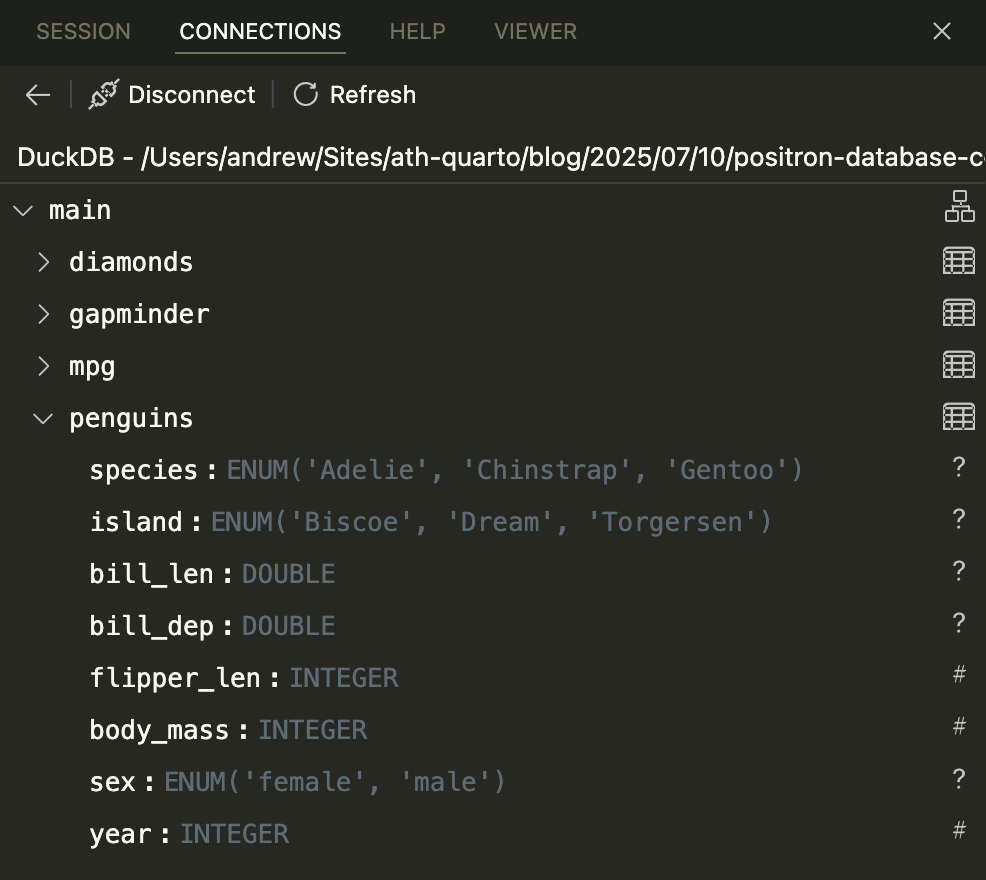
One more Positron-related post! It’s a quick one, just to highlight one feature I think is really neat and helpful: the Connections Pane. In a newer research project I’m working on, I have geocoded data for every foreign aid project run by most donor countries since 1989.