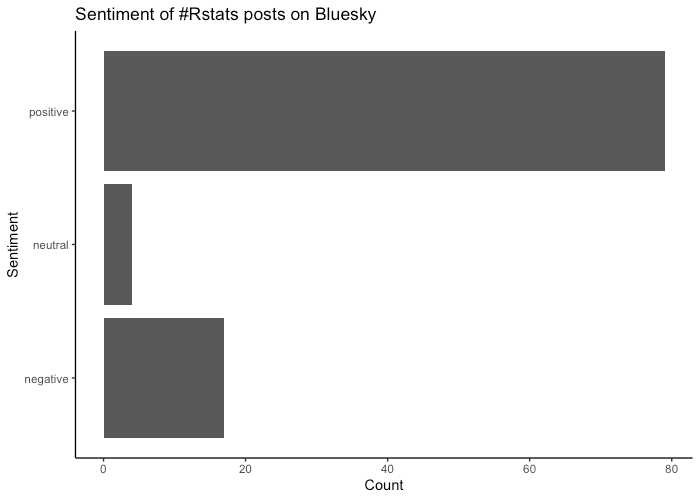
Background Bluesky, atrrr, local LLMs I’ve written a few posts lately about Bluesky — first, Bluesky for Science, about Bluesky as a home for Science Twitter expats after the mass eXodus, another on using the atrrr package to expand your Bluesky network. I’ve also spent some time looking at R packages to provide an interface to Ollama.