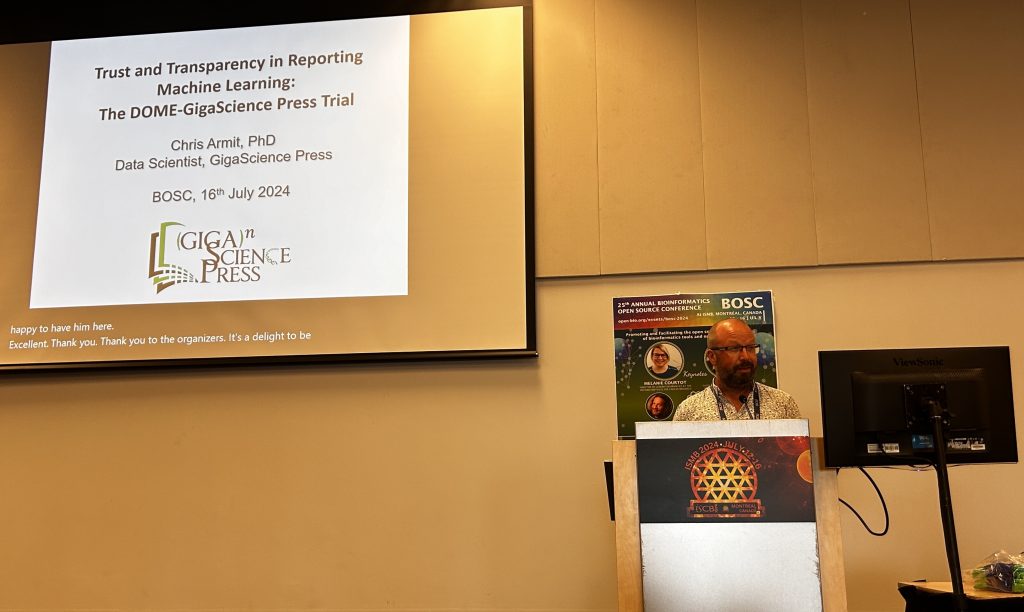
Watch a DOME Webinar on Machine Learning Best Practices & Recommendations on 24th September 2024 In recent years, there has been a substantial increase in scientific publications in journals publishing computational research such as ours utilising Machine Learning (ML). This represents a significant challenge for disseminating and assessing scientific research as the black box and […]
The post Machine Learning Standards in the Wild. DOME Webinar on ML Recommendations and Applications appeared first on GigaBlog.